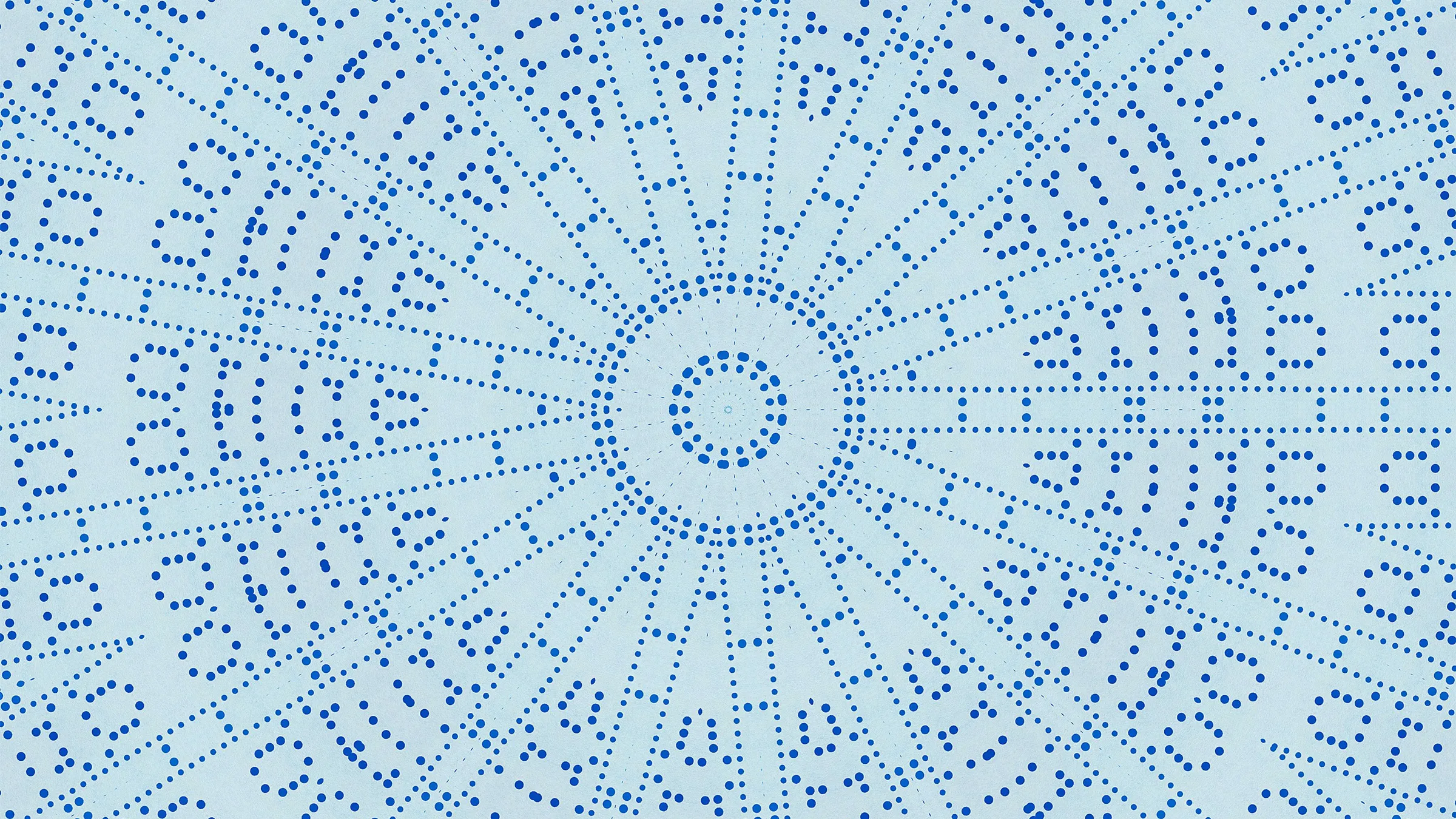
In the mid-1970s, AI research faced a crisis so severe that it almost brought the entire field to a standstill. This period, known as the First AI Winter, offers crucial lessons for today’s booming AI industry. As AI continues to dominate headlines and transform industries, it’s essential to reflect on the past to avoid repeating the same mistakes.
Causes of the First AI Winter
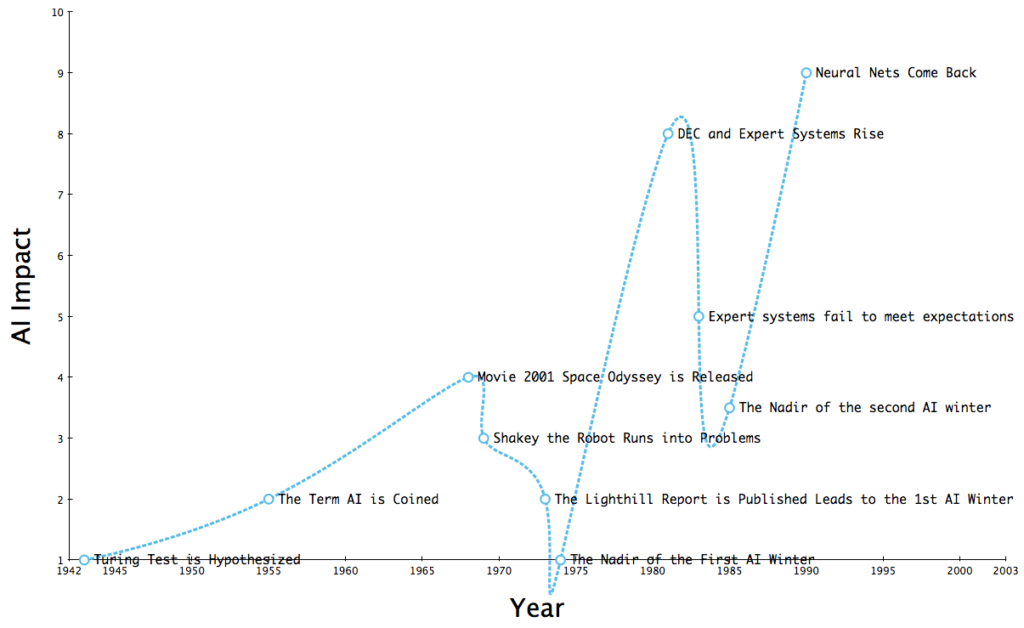
The First AI Winter was precipitated by a combination of overhyped expectations, technical limitations, and economic factors. Early AI researchers made bold predictions about imminent breakthroughs. For instance, Marvin Minsky once claimed that a machine with the intelligence of an average human being was just around the corner. However, these promises often fell short due to the complexities of AI problems such as word-sense disambiguation and combinatorial explosion.
The Lighthill Report and Criticism
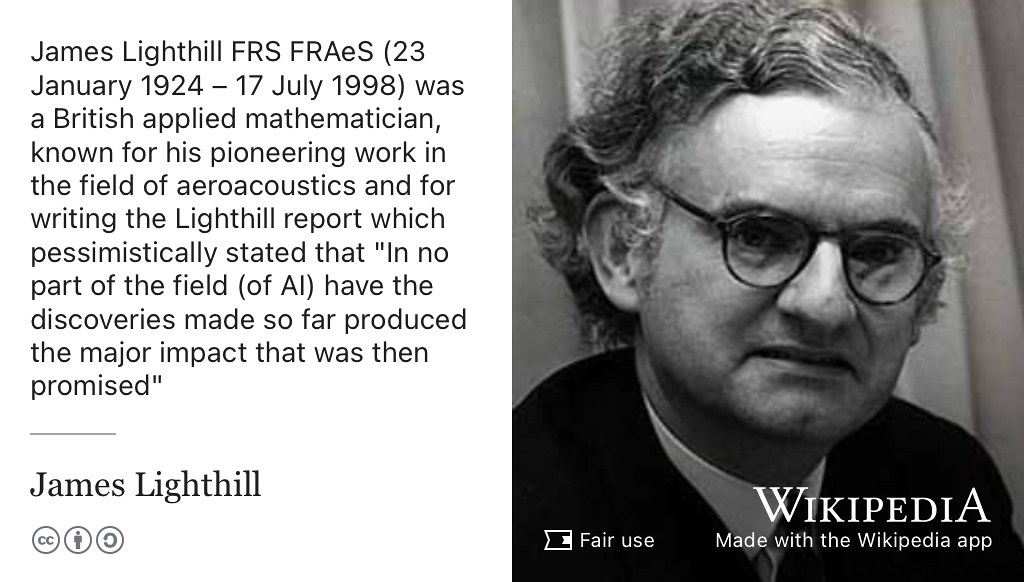
The publication of the Lighthill Report in 1973 was particularly damaging. It criticized AI’s progress and led to significant funding cuts in the UK. Sir James Lighthill’s report concluded that AI research had failed to achieve its “grandiose objectives” and highlighted the intractability of many AI algorithms when applied to real-world problems. This report didn’t just criticize; it reshaped the entire landscape of AI funding in the UK, causing a ripple effect that would be felt for years.
Economic Downturn
The economic downturn of the late 1970s also played a role, as budget cuts forced many research programs to shut down. Additionally, the high cost and limited performance of specialized AI hardware like Lisp machines, compared to more affordable and efficient general-purpose computers, further contributed to the decline in support for AI research. The global economic downturn forced governments and private sectors to tighten their belts, with high-cost AI research often being the first to face cuts.
Impact of the First AI Winter
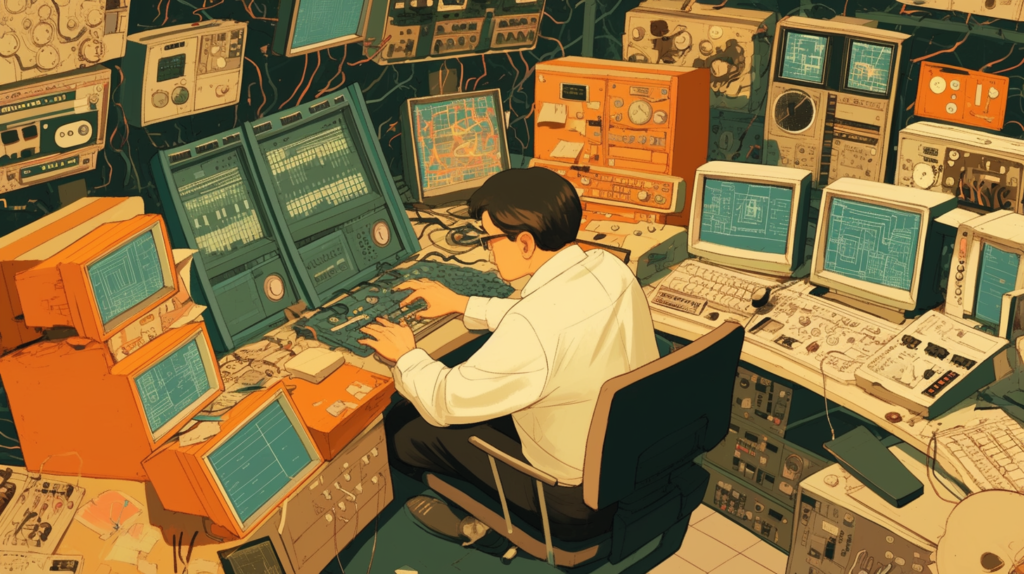
The First AI Winter resulted in widespread project cancellations, funding cuts, and a shift in focus away from AI. Many researchers left the field, leading to a significant slowdown in AI advancements. This period underscored the importance of managing expectations and the need for practical applications of AI technology to sustain interest and investment.
Key Figures and Their Contributions
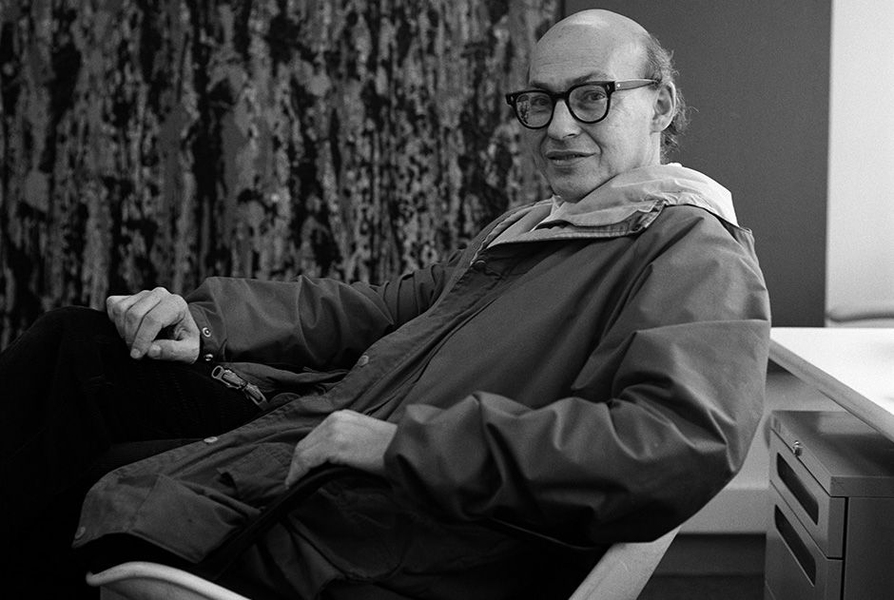
Despite the challenges, several key figures continued to shape AI during this period:
- Marvin Minsky: His skepticism about neural networks underscored the need for alternative approaches and highlighted the limitations of early AI systems. His famous debates with proponents of neural networks are legendary in the AI community.
- James Lighthill: His critical report reshaped funding priorities, casting a shadow over AI research.
- Herbert Simon: Despite his optimistic predictions, his work serves as a caution against overpromising.
- Arthur Samuel and John McCarthy: Their foundational work in AI laid the groundwork for future advancements. McCarthy’s development of the LISP programming language remains influential to this day.
Lessons for Today’s AI Landscape
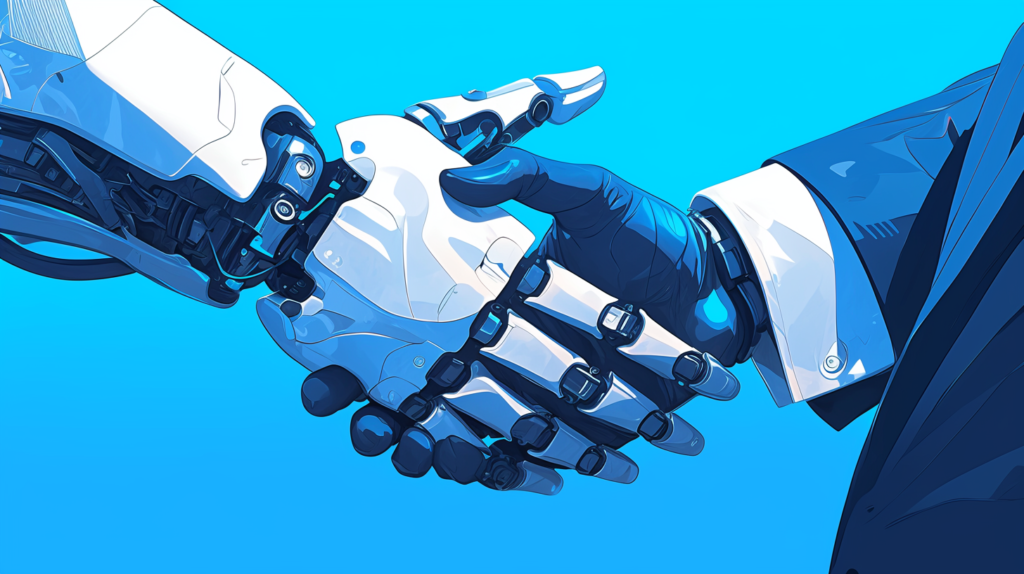
From the First AI Winter, we learn the importance of setting realistic expectations, acknowledging technical limitations, and promoting transparency in AI research. Today’s AI boom mirrors the past in its rapid advancements and high expectations, but we must balance optimism with practicality. Ensuring that AI technologies are transparent, explainable, and ethically sound is crucial to maintaining public trust and avoiding another downturn.
Parallels with Today’s AI Boom
Today’s AI landscape, with its emphasis on machine learning and neural networks, echoes the early optimism of the 1970s. As we see AI integrated into everything from healthcare to autonomous vehicles, it’s tempting to believe we’re on the verge of a utopia. However, the lessons from the First AI Winter remind us to be cautious of overhyping capabilities and to prepare for potential technical and ethical challenges. By learning from past mistakes, we can foster a more sustainable and responsible AI evolution.
Hypotheses for the Future: The Hypothetical Ceiling
A growing number of researchers are hypothesizing a hypothetical ceiling for the current AI boom, suggesting that we might either continue to see rapid growth in AI capabilities or hit a ceiling that prevents us from achieving Artificial General Intelligence (AGI). This could lead to another AI winter if users become disheartened by the perceived stagnation. While some experts believe AGI could emerge within a decade, others argue that significant theoretical and practical barriers remain, potentially delaying AGI for decades or more.
Key challenges include achieving true common sense reasoning, robust natural language understanding, and the ability to generalize knowledge across different domains. Without significant breakthroughs in these areas, AI might struggle to move beyond narrow applications, leading to unmet expectations and a possible downturn in enthusiasm and funding.
Revival Post-Winter Projects
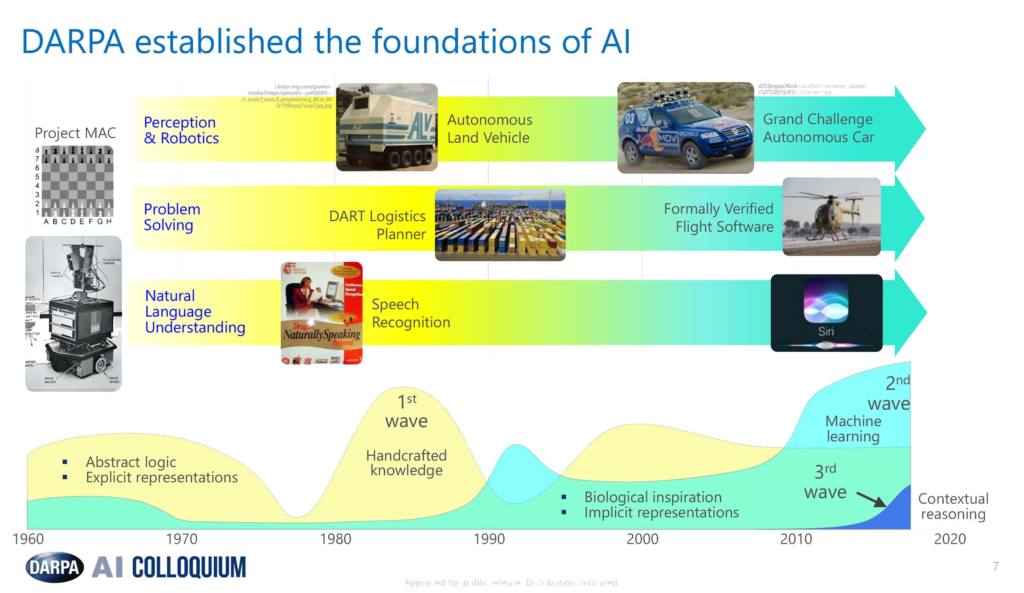
The end of the First AI Winter saw a resurgence in AI research through the development of expert systems and renewed DARPA funding. These efforts were bolstered by advancements in computing power and data availability, enabling more complex AI systems. Just as expert systems and renewed funding sparked a revival in the 1980s, today’s AI resurgence is powered by breakthroughs in deep learning and big data. Projects like OpenAI’s GPT-4o and Google’s AlphaGo illustrate the potential for AI to overcome previous limitations.
The First AI Winter serves as a cautionary tale for today’s AI boom. By understanding its causes and impacts, we can learn valuable lessons about setting realistic expectations, acknowledging technical limitations, and promoting transparency and accountability in AI research. As we move forward, let us strive for a responsible and sustainable approach to AI innovation, informed by the experiences of the past.
As we forge ahead in this exciting era of AI, let’s commit to learning from the past and fostering a future where AI innovation is both groundbreaking and responsible. Share your thoughts and join the conversation on how we can build a sustainable AI future.
By studying the First AI Winter, we can gain valuable insights into the challenges and pitfalls of AI research, ensuring a more sustainable and responsible approach to today’s AI advancements.